
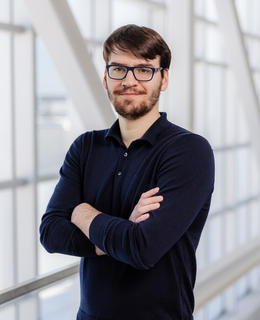
Dr. Roberto Souza
Positions
Associate Professor
Schulich School of Engineering, Department of Electrical and Software Engineering
Full Member
Hotchkiss Brain Institute
Associate Member
McCaig Institute for Bone and Joint Health
Contact information
Phone number
office: 403.210.6544
Background
Educational Background
PhD Computer Engineering, University of Campinas (UNICAMP), 2017
MSc Computer Engineering, UNICAMP, 2014
BSc Electrical Engineering, Federal University of Pará, 2012
Biography
Roberto Souza is a dual citizen from Brazil and the United States, working as an Assistant Professor at the Electrical and Software Engineering Department at the University of Calgary, Canada, since July 2020. He has a B.Sc. in Electrical Engineering from the Federal University of Pará (2012), an M.Sc. (2014) and PhD (2017), both in Computer Engineering from the University of Campinas (UNICAMP). Before becoming an Assistant Professor, Roberto worked for three years as a postdoctoral scholar in the Radiology Department at the University of Calgary. He has international experience, having worked as an intern at the Grenoble Institute of Technology, France, and the University of Pennsylvania, United States. Dr Souza has extensive expertise in image processing and machine learning. His research is currently focused on innovative strategies for data integration and data mining in imaging applications. Look at his Google Scholar page for a list of relevant publications.
Research
Areas of Research
Participation in university strategic initiatives
Courses
Course number | Course title | Semester |
---|---|---|
ENEL 645 | Data Mining & Machine Learning | Fall 2024 |
ENEL 645 | Data Mining & Machine Learning | Winter 2024 |
ENEL 645 | Data Mining & Machine Learning | Winter 2023 |
ENSF 619.2 | Advanced Topics in Image Analysis and Machine Learning | Winter 2023 |
ENDG 233 | Programming with Data | Fall 2022 |
ENEL 645 | Data Mining & Machine Learning | Winter 2022 |
ENSF 619.2 | Advanced Topics in Image Analysis and Machine Learning | Fall 2021 |
ENEL 645 | Data Mining & Machine Learning | Winter 2021 |
Awards
- Teaching Excellence, Schulich School of Engineering. 2023
- Early Research Excellence, Schulich School of Engineering. 2022
- UNICAMP Inventor’s Award, University of Campinas (UNICAMP). 2016
- Engineering Kawaguchi Award , Albras S.A.. 2012
Publications
Are you the profile owner?
Login to edit.