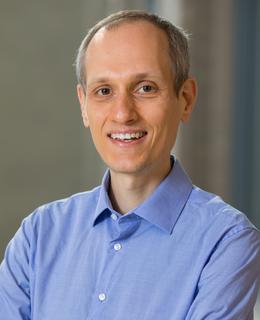
Dr. Matthias Wilms
Positions
Assistant Professor
Cumming School of Medicine, Department of Pediatrics
Assistant Professor
Cumming School of Medicine, Department of Community Health Sciences
Assistant Professor
Cumming School of Medicine, Department of Radiology
Child Health & Wellness Researcher
Alberta Children's Hospital Research Institute
Full Member
Hotchkiss Brain Institute
Background
Educational Background
PostDoc Medical Image Analysis, University of Calgary,
Dr. rer. nat. (German PhD equivalent) Computer Science, University of Luebeck, Germany,
MSc Computer Science, University of Hamburg, Germany,
BSc Applied Computer Science, Hamburg University of Applied Sciences, Germany,
Biography
Dr. Matthias Wilms is an Assistant Professor at the University of Calgary in the Departments of Paediatrics and Community Health Sciences. He received his BSc in Applied Computer Science from the Hamburg University of Applied Sciences in 2009, a MSc in Computer Science from the University of Hamburg in 2012, and his PhD in Computer Science from the University of Luebeck in 2018. Prior to joining the University of Calgary as a faculty member in 2022, he was a Postdoctoral Fellow in the Medical Image Processing and Machine Learning Lab at UCalgary. Dr. Wilms is an expert in machine learning-based medical image analysis. His research centers around the development of machine learning solutions for health data science and precision medicine problems where he is specifically interested in developing and advancing machine learning methods that accurately model the complex dynamics and variations of normal or pathological processes in the human body by integrating and combining diverse, large-scale medical data (e.g., images, clinical data, text reports). These models can then serve as clinical computer-aided diagnosis support tools or as tools for systematic data exploration in research scenarios. While the sensitivity and specificity of the models is of paramount importance in healthcare, his work also explicitly focuses on the explainability and interpretability of their decisions to enhance acceptability and trust by clinicians and patients. Finally, he is also interested in developing methods that achieve good results even if trained with limited data, which is a major problem in pediatric applications and/or rare diseases where data is especially challenging to collect. Examples of his work include the modeling of brain aging using neuroimaging data and the detection of genetic syndromes from 3D facial scans.
Research
Areas of Research
Participation in university strategic initiatives
Publications
Are you the profile owner?
Login to edit.