
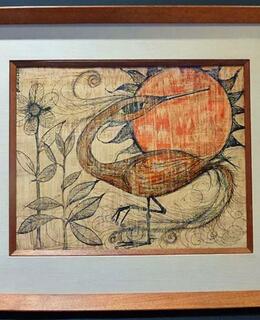
Alexander De Leon
Positions
Associate Professor
Faculty of Science, Department of Mathematics and Statistics
Contact information
Background
Educational Background
PhD Statistics , University of Alberta, 2002
Biography
Originally from the Philippines, Alexander R. de Leon received his PhD in Statistics from the University of Alberta. He is currently an associate professor in the Department of Mathematics and Statistics at the University of Calgary, where he enjoys teaching a wide variety of courses. He is interested in all aspects of statistics and likes to think about them while binge-streaming his favourite shows. He was an Associate Editor of the Journal of Statistical Computation & Simulation (2013-2021) and is currently a Topics Editor of Research Methods in Medicine & Health Sciences (since 2020). His research is supported by the Natural Sciences & Engineering Research Council (NSERC) of Canada. He served as Co-Chair (with Dr. Karen Kopciuk) of Local Arrangements Committee for 2019 Statistical Society of Canada Anual Meeting at the University of Calgary. He was also the WNAR-IBS Representative on the Program Committee for 2015 Joint Statistical Meetings in Seattle, WA.
Research
Areas of Research
Participation in university strategic initiatives
Courses
Course number | Course title | Semester |
---|---|---|
STAT 421 | Mathematical Statistics | Fall 2024 |
STAT 525/625 | Applied Multivariate Analysis/Multivariate Analysis | Fall 2024 |
DATA 602-01 | Statistical Data Analysis | Winter 2025 |
Awards
- GREAT Supervisor Award, Faculty of Graduate Studies, University of Calgary. 2014
- TUBITAK Visiting Scientist, Middle East Technical University, Government of Turkey. 2013
- Visiting Professor, School of Statistics, University of the Philippines-Diliman. 2017
- 2003 Joint Statistical Meetings Best Student Paper, Health Policy Statistics Section, American Statistical Association. 2003
- Pundit RD Sharma Memorial Graduate Award in Mathematical and Statistical Sciences, Department of Mathematical and Statistical Sciences, University of Alberta. 1999
- Honour Society of Phi Kappa Phi, University of the Philippines. 1991
Publications
- Measurement error correction in mediation analysis under the additive hazards model. Y. Yan, M. Ren & A. R. de Leon. Communications in Statistics - Simulation and Computation. 5083-5099. (2024)
- A two-level copula joint model for joint analysis of longitudinal and competing risks data. X. Lu, T. Chekouo, H. Shen, A. R. de Leon. Statistics in Medicine. 1909-1930. (2023)
- Model-based clustering and prediction with mixed measurements involving surrogate classifiers. H. Shen & A. R. de Leon. Statistics in Biopharmaceutical Research. 368-379. (2022)
- An empirical comparison of parametric and semiparametric time series regression models for overdispersed count data. M. Ghahramani, S. S. White & A. R. de Leon. Journal of Statistics and Management Systems. 879-905. (2022)
- Conditional dependence in longitudinal data analysis. M. Torabi & A. R. de Leon. Journal of the Iranian Statistical Society. 347-370. (2021)
- Binocular sensitivity and specificity of screening tests in cross-sectional diagnostic studies of paired organs. Y. Perera, M. Ren, J. R. B. Punzalan, C. J. Rudnisky, A. R. de Leon. Statistics in Medicine. 1754-1766. (2017)
- Performance of variable selection methods using stability-based selection. D. Lu, A. Weljie, A. R. de Leon, Y. McConnell, O. F. Bathe & K. Kopciuk. BMC Research Notes. Article Number 143. (2017)
- Gaussian copula distributions for mixed data, with application in discrimination. F. Jiryaie, N. Withanage, B. Wu, & A. R. de Leon. Journal of Statistical Computation and Simulation . 1643-1659. (2016)
- Joint estimation of multiple disease-specific sensitivities and specificities via crossed random effects models for correlated reader-based diagnostic data: Application of data cloning. N. Withanage, A. R. de Leon, C. J. Rudnisky. Statistics in Medicine. 3916-3928. (2015)
- Gaussian copula mixed models for clustered mixed outcomes, with application in developmental toxicology. B. Wu & A. R. de Leon. Journal of Agricultural, Biological, and Environmental Statistics. 39–56. (2014)
- Joint estimation of disease-specific sensitivities and specificities in reader-based multi-disease diagnostic studies of paired organs. N. Withanage, A. R. de Leon, C. J. Rudnisky. Journal of Applied Statistics. 2282-2297. (2014)
- Distributional results for L-statistics based on random vectors with multivariate elliptical distributions. A. R. de Leon, H. Mahmoodian, & A. Jamalizadeh. Chilean Journal of Statistics. 69-85. (2013)
- Copula-based regression models for a bivariate mixed discrete and continuous outcome. A. R. de Leon & B. Wu. Statistics in Medicine. 175-185. (2011)
- An elementary proof of independence of least squares estimation of regression coefficients and of variance in linear regression. A. R. de Leon & J. R. B. Punzalan. The Philippine Statistician. 77-81. (2013)
- Joint estimation of diagnostic accuracy measures for paired organs-application in ophthalmology. A. R. de Leon, A. Soo, D. C. Bonzo, & C. J. Rudnisky. Biometrical Journal. 837-850. (2009)
- ANOVA extensions for mixed discrete and continuous data. A. R. de Leon & Y. Zhu. Computational Statistics & Data Analysis. 2218-2227. (2008)
- General mixed-data model: Extension of general location and grouped continuous models. A. R. de Leon & K. C. Carriere. Canadian Journal of Statistics. 533-548. (2007)
- A generalized Mahalanobis distance for mixed data. A. R. de Leon & K. C. Carrière. Journal of Multivariate Analysis. 174-185. (2005)
- A likelihood approach to estimating sensitivity and specificity for binocular data: application in ophthalmology. A. R. de Leon, M. Guo, C. J. Rudnisky, & G. Singh. Statistics in Medicine. 3300-3314. (2007)
- One-sample likelihood ratio tests for mixed data. A. R. de Leon. Communications in Statistics - Theory and Methods . 129-141 . (2007)
- Mixed-outcome data. A. R. de Leon. Encyclopedia of Biopharmaceutical Statistics. 1414-1421. (2018)
- Analysis of Mixed Data: Methods & Applications. A R. de Leon & K. Carrière Chough. CRC/Chapman & Hall. (2013)
- Analysis of mixed data: An overview. A R. de Leon & K. Carrière Chough. Analysis of Mixed Data: Methods & Applications. 1-11. (2013)
- Joint analysis of mixed discrete and continuous outcomes via copulas. B. Wu, A. R. de Leon, & N. Withange. Analysis of Mixed Data: Methods & Applications. 139-156. (2013)
- Letter to the Editor re: Y. Kim, Y.-K. Choi, & S. Emery's "Logistic regression with multiple random effects: A simulation study of estimation methods and statistical packages". G. Inan, O. Ilk-Dag, & A. R. de Leon. The American Statistician. 129-130. (2014)
- Letter to the Editor re: T Sozu, T. Sugimoto, & T. Hamasaki's "Sample size determination in clinical trials with multiple co-primary endpoints including mixed continuous and binary variablesε". B. Wu & A. R. de Leon. Biometrical Journal. 807-812. (2013)
- Review of “Latent Markov Models for Longitudinal Data” by F. Bartolucci, A. Farcomeni, & F. Pennoni. A. R. de Leon. Technometrics. 139. (2015)
More Information
- Fahmida Yeasmin, Conditional Dependence in Joint Modelling and Analysis of Time-to-Event and Longitudinal Outcomes (Expected completion: Fall 2024, Co-supervisor: Dr. Hua Shen, University of Calgary).
- Joyce Raymund B. Punzalan, Joint Estimation of Diagnostic Accuracy Measures of Correlated Diagnostic Tests for Paired Organs in the Absence of a Gold Standard, School of Statistics, University of the Philippines-Diliman.
- Jia Li,, 2024, Correlated Data Analysis via EM Algorithm and Its Variants: Applications to Data on Physical Activity and Maternal Health. (Co-supervisor: Dr. Haocheng Li, Roche). Biostatistician, BC Centre for Disease Control, University of British Columbia.
- Mingchen Ren, 2022, Causal Inference with Mismeasured Confounders or Mediators. (Co-supervisor: Dr. Ying Yan, Sun Yat-sen University)
- Niroshan Withanage, 2013, Methods and Applications in the Analysis of Correlated Non-Gaussian Data. Senior Lecturer, Department of Statistics, University of Jayewardenepura, Colombo, Sri Lanka.
- Beilei Wu, 2013, Contributions to Copula Modelling of Mixed Discrete-Continuous Outcomes. Principal Biostatistician, PPD.
- Michael John Ilagan, 2020, A Goodness-of-Fit Test for the Bivariate Necessary-But-Not-Sufficient Relationship. (Co-Supervisor: Dr. Karen Kopciuk, Tom Baker Cancer Centre)
- Austin Mu Qing Ren, 2020, Analysis of Metabolomics Data via Mixed Models. (Co-Supervisor: Dr. Karen Kopciuk, Tom Baker Cancer Centre)
- Ajmery Jaman, 2019, Joint Modeling of Clustered Binary Data with Crossed Random Effects via the Gaussian Copula Mixed Model.
- Katherine L. Burak, 2019, Cluster Analysis of Correlated Non-Gaussian Continuous Data via Finite Mixtures of Gaussian Copula Distributions.
- Saifa Raz, 2016, COM-Poisson Clustering of Correlated Bivariate Over- and Under-Dispersed Counts.
- Mingchen Ren, 2016, Likelihood Analysis of Gaussian Copula Distributions for Mixed Data via a Parameter-Expanded Monte Carlo EM (PX-MCEM) Algorithm. (Co-supervisor: Dr. Ying Yan, Sun Yat-sen University)
- Mili Roy, 2016, Conditional Dependence in Joint Modelling of Longitudinal Non-Gaussian Outcomes.
- Ji, Ruan, 2015, Cluster Analysis of Gene Expression Profiles via Flexible Count Models for RNA-seq Data.
- Fahmida Yeasmin, 2015, Analysis of Serially Dependent Multivariate Longitudinal Non-Gaussian Continuous Data.
- Yamuni Singappuli Perera, 2013, Binocular Sensitivity and Specificity of Screening Tests in Prospective Studies of Paired Organs.
- Yifan Zhu, 2010, Evaluation of Binocular Screening Tests: A Copula Approach via "Continued" Binary Outcomes.
- Yongtao Zhu, 2006, ANOVA Extensions for Mixed Discrete and Continuous Data.
- Meiji Guo, 2005, A Likelihood-Based Approach to Estimating Sensitivity and Specificity with Binocular Diagnostic Data—Application in Ophthalmology.
Are you the profile owner?
Login to edit.